"Class" (Net Decoder)
NetDecoder["Class"]
represents a decoder that interprets a vector as class probabilities.
NetDecoder[{"Class",{c1,c2,…}}]
represents a decoder with class labels ci.
Details
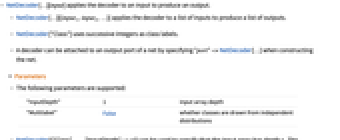
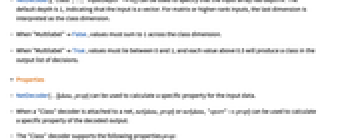
- NetDecoder[…][input] applies the decoder to an input to produce an output.
- NetDecoder[…][{input1,input2,…}] applies the decoder to a list of inputs to produce a list of outputs.
- NetDecoder["Class"] uses successive integers as class labels.
- A decoder can be attached to an output port of a net by specifying "port"->NetDecoder[…] when constructing the net.
- The following parameters are supported:
-
"InputDepth" 1 input array depth "Multilabel" False whether classes are drawn from independent distributions - NetDecoder[{"Class",…,"InputDepth"->n}] can be used to specify that the input array has depth n. The default depth is 1, indicating that the input is a vector. For matrix or higher-rank inputs, the last dimension is interpreted as the class dimension.
- When "Multilabel"False, values must sum to 1 across the class dimension.
- When "Multilabel"True, values must lie between 0 and 1, and each value above 0.5 will produce a class in the output list of decisions.
- NetDecoder[…][data,prop] can be used to calculate a specific property for the input data.
- When a "Class" decoder is attached to a net, net[data,prop] or net[data,"oport"->prop] can be used to calculate a specific property of the decoded output.
- The "Class" decoder supports the following properties prop:
-
"Decision" the class ci with the highest probability (default) "TopDecisions"n the n classes with the highest probabilities "TopProbabilities" probabilities for the most likely ci, returned as a list of rules "TopProbabilities"n probabilities for the n most likely ci "Probabilities" the association <c1->p1,c2->p2,… > "Probability"ci probability for a specific ci "Entropy" the entropy of the probability distribution "RandomSample" sample each class proportionally to its probability "RandomSample"t sample using a positive temperature t "RandomSample"{param1val1,…} random sampling with specific behavior None bypass decoding and return the input - Possible setting for parami in "RandomSample"{param1val1,…} include:
-
"Temperature"t sample using a positive temperature t "TotalProbabilityCutoff"p sample among the most probable classes with an accumulated probability of at least p (nucleus sampling) "TopProbabilities"k sample only among the k highest-probability classes
Parameters
Properties
Examples
open allclose allBasic Examples (1)
Scope (3)
Decoders are most commonly used by attaching them to the output of a net:
This allows the net to return a class label:
Create and attach a class decoder that uses successive integers as class labels:
Use it on a probability vector to make a class prediction:
Return the top two predictions:
Return the probabilities for all classes:
Return the entropy of the distribution: