SARMAProcess
SARMAProcess[{a1,…,ap},{b1,…,bq},{s,{α1,…,αm},{β1,…,βr}},v]
represents a weakly stationary seasonal autoregressive moving-average process with ARMA coefficients ai and bj, seasonal order s, seasonal ARMA coefficients αi and βj, and normal white noise with variance v.
SARMAProcess[{a1,…,ap},{b1,…,bq},{s,{α1,…,αm},{β1,…,βr}},Σ]
represents a weakly stationary vector SARMA process driven by normal white noise, with covariance matrix Σ.
SARMAProcess[{a1,…,ap},{b1,…,bq},{{s1,…},{α1,…,αm},{β1,…,βr}},Σ]
represents a weakly stationary vector SARMA process with multiple seasonal orders si.
SARMAProcess[{a1,…,ap},{b1,…,bq},{s,{α1,…,αm},{β1,…,βr}},v,init]
represents a SARMA process with initial data init.
SARMAProcess[c,…]
represents a SARMA process with a constant c.
Details
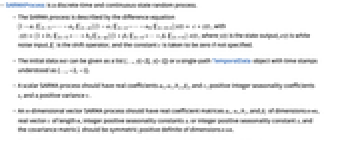
- SARMAProcess is a discrete-time and continuous-state random process.
- The SARMA process is described by the difference equation
, with
, where
is the state output,
is white noise input,
is the shift operator, and the constant c is taken to be zero if not specified.
- The initial data init can be given as a list {…,y[-2],y[-1]} or a single-path TemporalData object with time stamps understood as {…,-2,-1}.
- A scalar SARMA process should have real coefficients ai, αi, bj, βj, and c, positive integer seasonality coefficients s, and a positive variance v.
- An
-dimensional vector SARMA process should have real coefficient matrices ai, αi, bj, and βj of dimensions
×
, real vector c of length
, integer positive seasonality constants si or integer positive seasonality constant s, and the covariance matrix Σ should be symmetric positive definite of dimensions
×
.
- The SARMA process with zero constant has transfer function
, where
,
,
,
, and
is an n-dimensional unit.
- SARMAProcess[p,q,{s,sp,sq}] represents a SARMA process with autoregressive and moving-average orders p and q, their seasonal counterparts sp and sq, and seasonality s for use in EstimatedProcess and related functions.
- SARMAProcess can be used with such functions as CovarianceFunction, RandomFunction, and TimeSeriesForecast.
Examples
open allclose allBasic Examples (3)
Scope (33)
Basic Uses (9)
Simulate an ensemble of paths:
Simulate with given precision:
Simulate a scalar process with different seasonalities:
Sample paths for positive and negative values of the parameter:
Simulate a weakly stationary process with given initial values:
For a process with a trend, initial values influence the behavior of the whole path:
Simulate a two-dimensional process:
Create a 2D sample path function from the data:
The color of the path is the function of time:
Create a 3D sample path function with time:
The color of the path is the function of time:
Simulate a three-dimensional process:
Create a sample path function from the data:
The color of the path is the function of time:
Compare the sample correlation functions with that of the estimated process:
Use TimeSeriesModel to automatically find orders:
Compare the sample covariance functions with the best time series model:
Find the forecast for the next 20 steps:
Show the path of the forecast:
Plot the data and the forecasted values:
Find a forecast for a vector-valued time series process:
Covariance and Spectrum (5)
Closed-form correlation function for low order:
Covariance function for a vector-valued process:
Vector SARMAProcess:
Stationarity and Invertibility (4)
Estimation Methods (5)
The available methods for estimating a SARMAProcess:
Method of moments admits the following solvers:
This method allows for fixed parameters:
Some relations between parameters are also permitted:
Maximum conditional likelihood method allows the following solvers:
This method allows for fixed parameters:
Some relations between parameters are also permitted:
Maximum likelihood method allows the following solvers:
This method allows for fixed parameters:
Some relations between parameters are also permitted:
Spectral estimator allows you to specify windows used for PowerSpectralDensity calculation:
Spectral estimator allows following solvers:
Process Slice Properties (5)
Single time SliceDistribution:
Multiple time slice distributions:
Slice distribution of a vector-valued time series:
First-order stationary probability density function:
Compare with the density function of a normal distribution:
Compute the expectation of an expression:
CentralMoment and its generating function:
FactorialMoment has no closed form for symbolic order:
Cumulant and its generating function:
Representations (5)
Approximate with an ARProcess:
Compare the covariance function for the original and the approximate processes:
Approximate with an MAProcess:
Represent as equivalent ARMAProcess:
TransferFunctionModel representation:
StateSpaceModel representation:
Applications (3)
Use a SARMA process to model daily, monthly, and half-yearly autocorrelations:
The covariance function shows the serial correlations:
Daily mean temperature readings on the first of each month from the years 2000–2011 near your location:
The estimated time series process:
Forecast future values for the next three years:
Fit a SARMA model for the hourly measurements of temperature in August:
Properties & Relations (3)
SARMAProcess is a generalization of an ARMAProcess:
SARMAProcess is a generalization of an ARProcess:
SARMAProcess is a generalization of an MAProcess:
Possible Issues (2)
Some properties are defined only for weakly stationary processes:

Use FindInstance to find a weakly stationary process:
ToInvertibleTimeSeries does not always exist:

There are zeros of TransferFunctionModel lying on the unit circle:
Neat Examples (2)
Simulate a weakly stationary three-dimensional SARMAProcess:
Non-weakly stationary process, starting at the origin:
Simulate paths from a SARMA process:
Take a slice at 50 and visualize its distribution:
Plot paths and histogram distribution of the slice distribution at 50:
Text
Wolfram Research (2012), SARMAProcess, Wolfram Language function, https://reference.wolfram.com/language/ref/SARMAProcess.html (updated 2014).
CMS
Wolfram Language. 2012. "SARMAProcess." Wolfram Language & System Documentation Center. Wolfram Research. Last Modified 2014. https://reference.wolfram.com/language/ref/SARMAProcess.html.
APA
Wolfram Language. (2012). SARMAProcess. Wolfram Language & System Documentation Center. Retrieved from https://reference.wolfram.com/language/ref/SARMAProcess.html