LearningRateMultipliers
Details
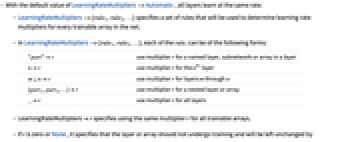
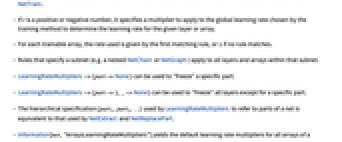
- With the default value of LearningRateMultipliers->Automatic, all layers learn at the same rate.
- LearningRateMultipliers->{rule1,rule2,…} specifies a set of rules that will be used to determine learning rate multipliers for every trainable array in the net.
- In LearningRateMultipliers->{rule1,rule2,…}, each of the rulei can be of the following forms:
-
"part"r use multiplier r for a named layer, subnetwork or array in a layer nr use multiplier r for the n layer
m;;nr use multiplier r for layers m through n {part1,part2,…}r use multiplier r for a nested layer or array _r use multiplier r for all layers - LearningRateMultipliersr specifies using the same multiplier r for all trainable arrays.
- If r is zero or None, it specifies that the layer or array should not undergo training and will be left unchanged by NetTrain.
- If r is a positive or negative number, it specifies a multiplier to apply to the global learning rate chosen by the training method to determine the learning rate for the given layer or array.
- For each trainable array, the rate used is given by the first matching rule, or 1 if no rule matches.
- Rules that specify a subnet (e.g. a nested NetChain or NetGraph) apply to all layers and arrays within that subnet.
- LearningRateMultipliers->{part->None} can be used to "freeze" a specific part.
- LearningRateMultipliers->{part->1,_->None} can be used to "freeze" all layers except for a specific part.
- The hierarchical specification {part1,part2,…} used by LearningRateMultipliers to refer to parts of a net is equivalent to that used by NetExtract and NetReplacePart.
- Information[net,"ArraysLearningRateMultipliers"] yields the default learning rate multipliers for all arrays of a net.
- The multipliers that are genuinely used when training can be obtained from a NetTrainResultsObject via the property "ArraysLearningRateMultipliers".
Examples
open allclose allBasic Examples (2)
Create and initialize a net with three layers, but train only the last layer:
Evaluate the trained net on an input:
The first layer of the initial net started with zero biases:
The biases of the first layer remain zero in the trained net:
The biases of the third layer have been trained:
Create a frozen layer with given array values:
Nest this layer inside a bigger net:
Get the learning rate multipliers that will be used by default in NetTrain, for all arrays of the net:
Check the learning rate multipliers that were used to train:
The arrays of the frozen layer were unchanged during training:
Scope (1)
Replace LearningRateMultipliers in a Network (1)
Set the LearningRateMultipliers of the first layer of this net to zero:
Check programmatically the values of LearningRateMultipliers options:
Applications (1)
Train an existing network to solve a new task. Obtain a pre-trained convolutional model that was trained on handwritten digits:
Remove the final two layers, and attach two new layers, in order to classify images into 3 classes:
Generate training data by rasterizing the characters "x", "y", and "z" with a variety of fonts, sizes, and cases:
Train the modified network on the new task:
Measure the performance on the original training data, which includes the training and validation set:
Properties & Relations (1)
Train LeNet on the MNIST dataset with specific learning rate multipliers, returning a NetTrainResultsObject:
Obtain the actual learning rate multipliers used on individual weight arrays:
Possible Issues (1)
When a shared array occurs at several places in the network, only a unique learning rate multiplier will be applied to all the occurrences of the shared array.
Create a network with shared arrays:
Specifying a LearningRateMultipliers to a shared array in the network will assign the same multiplier to all places:
If there is a conflict, the first matching value will be used:
The same happens when LearningRateMultipliers is specified when constructing the network:
Text
Wolfram Research (2017), LearningRateMultipliers, Wolfram Language function, https://reference.wolfram.com/language/ref/LearningRateMultipliers.html (updated 2020).
CMS
Wolfram Language. 2017. "LearningRateMultipliers." Wolfram Language & System Documentation Center. Wolfram Research. Last Modified 2020. https://reference.wolfram.com/language/ref/LearningRateMultipliers.html.
APA
Wolfram Language. (2017). LearningRateMultipliers. Wolfram Language & System Documentation Center. Retrieved from https://reference.wolfram.com/language/ref/LearningRateMultipliers.html