ActivePrediction
ActivePrediction[f,{conf1,conf2, …}]
给出一个对象,表示通过使用函数 f 来确定实例配置 confi 的值而获得的主动预测的结果.
ActivePrediction[f,reg]
在由 reg 指定的区域内产生配置.
ActivePrediction[f,sampler]
通过应用函数 sampler 产生配置.
ActivePrediction[f,{conf1,conf2,…}nsampler]
应用函数 nsampler 以连续产生从某个 confi 开始的配置.
更多信息和选项
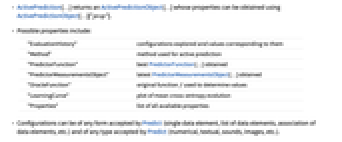
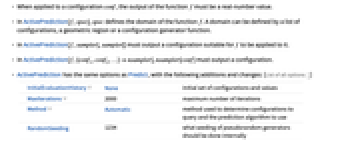
- ActivePrediction[…] 返回 ActivePredictionObject[…],可以用 ActivePredictionObject[…]["prop"] 来获取它的属性.
- 可能的属性包括:
-
"EvaluationHistory" 尝试过的配置和相应的值 "Method" 用于主动预测的方法 "PredictorFunction" 得到的最佳 PredictorFunction[…] "PredictorMeasurementsObject" 得到的最新 PredictorMeasurementsObject[…] "OracleFunction" 用于确定值的原始函数 f "LearningCurve" 平均交叉熵演化图 "Properties" 所有可用属性列表 - 配置可以是 Predict 接受的任意形式(单个数据元素、数据元素列表、数据元素关联等)和 Predict 接受的任意类型(数值、文字、声音、图像等类型).
- 当应用于配置 conf 时,函数 f 的输出必须是实数.
- 在 ActivePrediction[f,spec] 中,spec 定义了函数 f 的域. 可以用配置列表、几何区域或配置产生函数来定义域.
- 在 ActivePrediction[f,sampler] 中,sampler[] 必须输出一个适宜于 f 的应用的配置.
- 在 ActivePrediction[f,{conf1,conf2,…}nsampler] 中,nsampler[conf] 必须输出一个配置.
- ActivePrediction 和 Predict 有同样的选项,更多选项及不同之处如下所示: [所有选项的列表]
-
InitialEvaluationHistory None 初始配置和数值 MaxIterations 2000 最大迭代次数 Method Automatic 用来确定用于查询和预测算法的配置的方法 RandomSeeding 1234 伪随机发生器应该在内部完成的种子生成方式 - Method 的可能设置包括:
-
Automatic 自动选择方法 "Randomized" 从域中随机选择配置 "MaxEntropy" 选择预测器具有最大不确定性的配置 assoc 指定计算策略和预测方法的关联 - 在 Methodassoc 形式中,关联的元素为:
-
"EvaluationStrategy" 用来确定用于查询的配置的方法 "PredictionMethod" 预测时使用的方法 - RandomSeeding 的可能设置包括:
-
Automatic 每次调用函数时都会自动重新设置种子 Inherited 使用外部种子随机数 seed 使用明确的整数或字符串作为种子 -
AcceptanceThreshold Automatic 异常检测器的稀有概率阈值 AnomalyDetector None 预测器使用的异常检测器 FeatureExtractor Identity 如何提取要学习的特征 FeatureNames Automatic 特征应具备什么名称 FeatureTypes Automatic 对输入数据所假定的特征类型 IndeterminateThreshold 0 在哪个概率密度之下返回 Indeterminate InitialEvaluationHistory None 初始配置和数值 MaxIterations 2000 最大迭代次数 Method Automatic 用来确定用于查询和预测算法的配置的方法 MissingValueSynthesis Automatic 如何合成缺失值 PerformanceGoal Automatic 具有某种优点的算法 RandomSeeding 1234 伪随机发生器应该在内部完成的种子生成方式 RecalibrationFunction Automatic 如何对预测值进行后处理 TargetDevice "CPU" 要在其上执行训练的目标设备 TimeGoal Automatic 花多长时间来训练分类器 TrainingProgressReporting Automatic 如何报告训练时的进展 UtilityFunction Automatic 被表示为实际和预测值的函数的效用 ValidationSet Automatic 用于验证所产生模型的数据
所有选项的列表
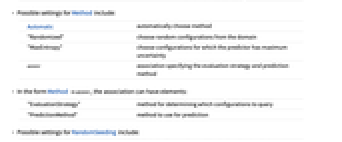
范例
打开所有单元关闭所有单元基本范例 (3)
培训一个 ActivePredictionObject[…],找到一个给定配置集的函数的预测器:
训练一个预测对象,找到 Det 函数的预测器,其中域由配置生成器定义:
选项 (3)
InitialEvaluationHistory (1)
应用 (2)
LogLikelihood 函数预测器 (1)
加载 Fisher 的 Iris 数据集并把它分为培训集和测试集:
构建一个 LogLikelihood 函数,它在培训样本上培训一个分类器,然后对于给定的超参数选择在测试样本上给出 "LogLikelihoodRate":
培训一个预测对象,找到长方形域上的 LogLikelihood 函数的预测器:
文本
Wolfram Research (2017),ActivePrediction,Wolfram 语言函数,https://reference.wolfram.com/language/ref/ActivePrediction.html (更新于 2017 年).
CMS
Wolfram 语言. 2017. "ActivePrediction." Wolfram 语言与系统参考资料中心. Wolfram Research. 最新版本 2017. https://reference.wolfram.com/language/ref/ActivePrediction.html.
APA
Wolfram 语言. (2017). ActivePrediction. Wolfram 语言与系统参考资料中心. 追溯自 https://reference.wolfram.com/language/ref/ActivePrediction.html 年