GARCHProcess
GARCHProcess[κ,{α1,…,αq},{β1,…,βp}]
represents a generalized autoregressive conditionally heteroscedastic process of orders p and q, driven by a standard white noise.
GARCHProcess[κ,{α1,…,αq},{β1,…,βp},init]
represents a GARCH process with initial data init.
Details
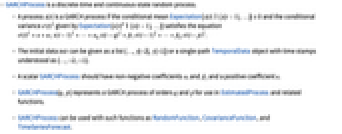
- GARCHProcess is a discrete-time and continuous-state random process.
- A process x[t] is a GARCH process if the conditional mean Expectation[x[t]{x[t-1],…}]=0 and the conditional variance
given by Expectation[x[t]2{x[t-1],…}] satisfies the equation
.
- The initial data init can be given as a list {…,y[-2],y[-1]} or a single-path TemporalData object with time stamps understood as {…,-2,-1}.
- A scalar GARCHProcess should have non-negative coefficients αi and βj and a positive coefficient κ.
- GARCHProcess[q,p] represents a GARCH process of orders q and p for use in EstimatedProcess and related functions.
- GARCHProcess can be used with such functions as RandomFunction, CovarianceFunction, and TimeSeriesForecast.
Examples
open allclose allBasic Examples (3)
Simulate a GARCHProcess:
Unconditional mean and variance of a weakly stationary process:
Scope (13)
Basic Uses (8)
Simulate an ensemble of paths:
Simulate with arbitrary precision:
Simulate a weakly stationary process with given initial values:
A non-weakly stationary process:
An integrated GARCHProcess:
Explosive GARCHProcess:
Such a process is not second-order stationary:
Conditions for a GARCHProcess to be covariance-stationary:
Region of second-order stationarity for a GARCHProcess[1,1]:
Estimate a GARCHProcess:
Use maximum conditional likelihood:
Find the forecast 20 steps ahead:
Find the mean squared errors of the forecast:
The forecasted states are equal to zero, hence the forecasted standard deviation bounds are:
Process Slice Properties (5)
Moments of a weakly stationary GARCH of orders :
Moment of a GARCH process with given initial conditions:
Region where kurtosis is defined:
Probability density function of the sample:
Use the Monte Carlo method to calculate NProbability for slice distribution:
Calculate NExpectation:
Compare to the second Moment:
Properties & Relations (3)
The values of a GARCHProcess are uncorrelated:
Corresponding ARMAProcess:
For a process with given initial values:
Squared values of a GARCHProcess follow an ARMAProcess:
CorrelationFunction and PartialCorrelationFunction of squared values:
The corresponding ARMA process:
CorrelationFunction and PartialCorrelationFunction of the ARMA process:
Text
Wolfram Research (2014), GARCHProcess, Wolfram Language function, https://reference.wolfram.com/language/ref/GARCHProcess.html.
CMS
Wolfram Language. 2014. "GARCHProcess." Wolfram Language & System Documentation Center. Wolfram Research. https://reference.wolfram.com/language/ref/GARCHProcess.html.
APA
Wolfram Language. (2014). GARCHProcess. Wolfram Language & System Documentation Center. Retrieved from https://reference.wolfram.com/language/ref/GARCHProcess.html