TensorRank
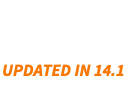
TensorRank[tensor]
gives the rank of tensor.
Details and Options

- TensorRank accepts any type of tensor, either symbolic or explicit, including any type of array.
- On explicit rectangular arrays of scalars, TensorRank coincides with ArrayDepth. On symbolic arrays, TensorRank stays unevaluated unless the array has been assigned a rank through any form of assumption.
Examples
open allclose allBasic Examples (4)
Scope (4)
Options (2)
GenerateConditions (1)
By default, TensorRank quietly makes assumptions necessary for the input to be well-defined:
With GenerateConditionsTrue, TensorRank gives a conditional result:
With GenerateConditionsNone, TensorRank fails when assumptions are necessary:

Properties & Relations (2)
On explicit arrays, TensorRank coincides with ArrayDepth:
Possible Issues (3)
TensorRank can obtain some information contextually. Expressions without tensor properties inside numeric functions, arrays, or derivatives are considered scalars:
It is not possible to mix incompatible local and global assumptions:

TensorRank does not check for dimensions homogeneity, only rank homogeneity:

With GenerateConditionsTrue, TensorRank checks for dimensions homogeneity:

Text
Wolfram Research (2012), TensorRank, Wolfram Language function, https://reference.wolfram.com/language/ref/TensorRank.html (updated 2024).
CMS
Wolfram Language. 2012. "TensorRank." Wolfram Language & System Documentation Center. Wolfram Research. Last Modified 2024. https://reference.wolfram.com/language/ref/TensorRank.html.
APA
Wolfram Language. (2012). TensorRank. Wolfram Language & System Documentation Center. Retrieved from https://reference.wolfram.com/language/ref/TensorRank.html