Linear Algebra Examples
Matrix Ordering | Finite Difference Solutions |
Full Rank Least Squares Solutions | Mesh Partitioning |
Minimization of 1 and Infinity Norms | Matrix Functions with NDSolve |
This tutorial shows a number of examples of the use of Wolfram Language for computations that involve linear algebra.
Matrix Ordering
Certain sparse matrix techniques try to reorder the matrix so that elements are grouped into blocks. The computation then works on each block using dense matrix techniques. One simple way to order a matrix into blocks involves sorting according to the sum of elements on each row. This will be demonstrated in this example.
Full Rank Least Squares Solutions
Solving a matrix equation is one of the key problems of matrix computation; techniques for solving it are discussed under "Solving Linear Systems". If
is an
×
matrix, this is a set of
linear equations in
unknowns. If
, there are more equations than unknowns and the system is overdetermined. A general technique for finding least squares solutions is given under "Least Squares Solutions". This example will show some different techniques for finding approximate solutions to overdetermined systems for full rank matrices.
Note that all the example solutions in this section would work just as well if they were given sparse matrices as input.
Least Squares Cholesky
This technique uses a Cholesky decomposition to find a least squares solution. If a matrix has full rank, the solution can be found in the following way.
This technique is fast, but is less accurate because it computes .
Least Squares QR
When is full rank, a more accurate way to solve the problem is to use the QR decomposition as follows.
Minimization of 1 and Infinity Norms
Many techniques for finding approximate solutions for the matrix equation when it is overdetermined (i.e., when
) work by minimizing the 2-norm. This is because of certain advantages that make it computationally tractable. One reason is that the function
is differentiable and differentiation is a linear operation. Thus, a linear system can be formed that finds the minimizing solutions. Another reason is that the 2-norm is preserved under orthogonal transformations. This means that a range of equivalent problems can be formed, which may be easier to solve.
However, there are other solutions that can be found by minimizing other norms, such as the 1-norm or the -norm. These may be more desirable in the particular context because they may find solutions that maintain important properties relevant to the individual problem. In this example techniques are shown to find approximate solutions that minimize these norms; both will use a method to find minimum values of constrained linear problems; typically this is known as linear programming.
In Wolfram Language, linear programming is provided by the function LinearProgramming. This can solve the linear programming problem for the different types of numbers that Wolfram Language supports: integers and rational numbers, as well as machine-precision approximate real and arbitrary-precision approximate real numbers. In addition, it provides techniques that are suitable for minimizing extremely large systems by means of an interior point method.
The solutions given in this section are suitable for dense matrix input. It would be straightforward to modify them for sparse matrix input; this would be necessary to take full advantage of the interior point linear programming technique.
Note that the techniques in this section could be extended to add other constraints on the solution.
One-Norm Minimization
Minimizing the 1-norm involves finding the value of that minimizes the following.
This is done by forming new variables and finding the minimum.
Infinity-Norm Minimization
Minimizing the -norm is similar to minimizing the 1-norm. It involves finding the value of
that minimizes the following.
This is done by forming new variables and finding the minimum.
Finite Difference Solutions
One way to solve partial differential equations is to form a spatial discretization and solve them with finite differences. This example considers a basic finite difference solution for the Poisson equation on a unit square.

Mesh Partitioning
This example demonstrates a practical use for extreme eigenvalues. The aim is to partition the vertices of a mesh/graph into two subdomains, with each part having roughly equal numbers of vertices and with the partition cutting through as few edges as possible. (An edge is cut if its two vertices lie in each of the two subdomains.) One way to partition a graph is to form the Laplacian and use its Fiedler vector (the eigenvector that corresponds to the second smallest eigenvalue) to order the vertices. There are more efficient algorithms for graph partitioning that do not require the calculation of the eigenvector, but the approach using Fiedler remains an important one.
Data
Plotting the Mesh
The Laplacian
A Laplacian of a graph, , is an
×
sparse matrix, with
the number of vertices in the graph. The entries of
are mostly zero, except that the diagonals are positive integers that correspond to the degree of the vertex, where the degree is the number of vertices linked to the vertex. In addition, if two vertices,
and
, are joined by an edge, the entry
is -1.
The Fiedler Vector
The sum of each row is zero, therefore the Laplacian matrix is positive semi-definite with a zero eigenvalue. The eigenvector corresponding to the second smallest eigenvalue is called the Fiedler vector.
Partitioning the Nodes
Matrix Functions with NDSolve
One of the important features of many of Wolfram Language's numerical solvers is that they work for vector and matrix input. Typically this is used to solve coupled systems of equations, but it can be used to solve matrix specific problems. One example will be given here that uses the numerical differential equation solver, NDSolve, to find parametrized solutions to matrix functions.
The exponential function is the solution of the differential equation.
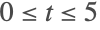