MeanAbsoluteLossLayer
represents a loss layer that computes the mean absolute loss between the "Input" port and "Target" port.
Details and Options
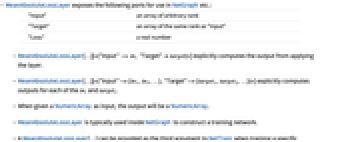
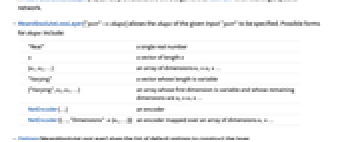
- MeanAbsoluteLossLayer exposes the following ports for use in NetGraph etc.:
-
"Input" an array of arbitrary rank "Target" an array of the same rank as "Input" "Loss" a real number - MeanAbsoluteLossLayer[…][<"Input" -> in, "Target"target >] explicitly computes the output from applying the layer.
- MeanAbsoluteLossLayer[…][<"Input"->{in1,in2,…},"Target"->{target1,target2,…} >] explicitly computes outputs for each of the ini and targeti.
- When given a NumericArray as input, the output will be a NumericArray.
- MeanAbsoluteLossLayer is typically used inside NetGraph to construct a training network.
- A MeanAbsoluteLossLayer[…] can be provided as the third argument to NetTrain when training a specific network.
- MeanAbsoluteLossLayer["port"->shape] allows the shape of the given input "port" to be specified. Possible forms for shape include:
-
"Real" a single real number n a vector of length n {n1,n2,…} an array of dimensions n1×n2×… "Varying" a vector whose length is variable {"Varying",n2,n3,…} an array whose first dimension is variable and whose remaining dimensions are n2×n3×… NetEncoder[…] an encoder NetEncoder[{…,"Dimensions"{n1,…}}] an encoder mapped over an array of dimensions n1×… - Options[MeanAbsoluteLossLayer] gives the list of default options to construct the layer. Options[MeanAbsoluteLossLayer[…]] gives the list of default options to evaluate the layer on some data.
- Information[MeanAbsoluteLossLayer[…]] gives a report about the layer.
- Information[MeanAbsoluteLossLayer[…],prop] gives the value of the property prop of MeanAbsoluteLossLayer[…]. Possible properties are the same as for NetGraph.
Examples
open allclose allBasic Examples (3)
Create a MeanAbsoluteLossLayer layer:
Create a MeanAbsoluteLossLayer that takes length-3 vectors:
Create a NetGraph containing a MeanAbsoluteLossLayer:
Scope (4)
Arguments (1)
Create a MeanAbsoluteLossLayer:
Apply the MeanAbsoluteLossLayer to a pair of matrices:
Apply the MeanAbsoluteLossLayer to a pair of vectors:
Apply the MeanAbsoluteLossLayer to a pair of numbers:
Ports (3)
Create a MeanAbsoluteLossLayer that assumes the input data are vectors of length 2:
Thread the layer across a batch of inputs:
Create a MeanAbsoluteLossLayer that takes two variable-length vectors:
Apply the layer to an input and target vector:
Thread the layer over a batch of input and target vectors:
Create a MeanAbsoluteLossLayer that takes two images as input:
Applications (1)
Define a single-layer neural network that takes in scalar numeric values and produces scalar numeric values, and train this network using a MeanAbsoluteLossLayer:
Properties & Relations (2)
MeanAbsoluteLossLayer computes:
Compare the output of the layer and the definition on an example:
MeanAbsoluteLossLayer effectively computes a normalized version of ManhattanDistance:
Text
Wolfram Research (2016), MeanAbsoluteLossLayer, Wolfram Language function, https://reference.wolfram.com/language/ref/MeanAbsoluteLossLayer.html (updated 2019).
CMS
Wolfram Language. 2016. "MeanAbsoluteLossLayer." Wolfram Language & System Documentation Center. Wolfram Research. Last Modified 2019. https://reference.wolfram.com/language/ref/MeanAbsoluteLossLayer.html.
APA
Wolfram Language. (2016). MeanAbsoluteLossLayer. Wolfram Language & System Documentation Center. Retrieved from https://reference.wolfram.com/language/ref/MeanAbsoluteLossLayer.html