NetInitialize
NetInitialize[net]
gives a net in which all uninitialized learnable parameters in net have been given initial values.
NetInitialize[net,All]
gives a net in which all learnable parameters have been given initial values.
Details and Options
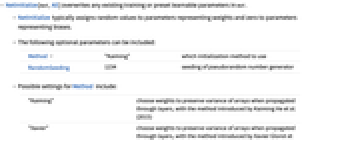
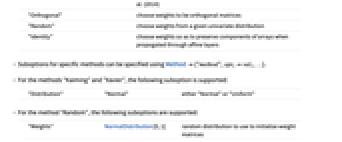
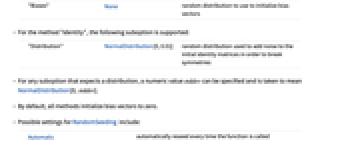
- NetInitialize[net,All] overwrites any existing training or preset learnable parameters in net.
- NetInitialize typically assigns random values to parameters representing weights and zero to parameters representing biases.
- The following optional parameters can be included:
-
Method "Kaiming" which initialization method to use RandomSeeding 1234 seeding of pseudorandom number generator - Possible settings for Method include:
-
"Kaiming" choose weights to preserve variance of arrays when propagated through layers, with the method introduced by Kaiming He et al. (2015) "Xavier" choose weights to preserve variance of arrays when propagated through layers, with the method introduced by Xavier Glorot et al. (2014) "Orthogonal" choose weights to be orthogonal matrices "Random" choose weights from a given univariate distribution "Identity" choose weights so as to preserve components of arrays when propogated through affine layers - Suboptions for specific methods can be specified using Method{"method",opt1val1,…}.
- For the methods "Kaiming" and "Xavier", the following suboption is supported:
-
"Distribution" "Normal" either "Normal" or "Uniform" - For the method "Random", the following suboptions are supported:
-
"Weights" NormalDistribution[0,1] random distribution to use to initialize weight matrices "Biases" None random distribution to use to initialize bias vectors - For the method "Identity", the following suboption is supported:
-
"Distribution" NormalDistribution[0,0.01] random distribution used to add noise to the initial identity matrices in order to break symmetries - For any suboption that expects a distribution, a numeric value stddev can be specified and is taken to mean NormalDistribution[0,stddev].
- By default, all methods initialize bias vectors to zero.
- Possible settings for RandomSeeding include:
-
Automatic automatically reseed every time the function is called Inherited use externally seeded random numbers seed use an explicit integer or strings as a seed
Examples
open allclose allBasic Examples (1)
Scope (1)
Options (1)
Properties & Relations (2)
NetTrain will automatically call NetInitialize before training begins. The weights and biases of a simple layer are initialized before training:
Extract the weights and biases after training:
Create a net that maps vectors of length 1 to vectors of length 1:
Initialize the net using the "Identity" method, which results in a net that attempts to preserve the components of arrays as they pass through linear layers:
Visualize the output of the net as a function of its input:
Initializing the net using other methods produces a random linear function:
Possible Issues (2)
Parameters belonging to certain layers have a fixed initialization method, independent of the Method option in NetInitialize:
By default, NetInitialize uses RandomSeeding1234, which will use the same random seed to initialize the net when NetInitialize is called repeatedly:
Use RandomSeedingAutomatic to ensure that repeated calls produce different initializations:
Text
Wolfram Research (2016), NetInitialize, Wolfram Language function, https://reference.wolfram.com/language/ref/NetInitialize.html (updated 2022).
CMS
Wolfram Language. 2016. "NetInitialize." Wolfram Language & System Documentation Center. Wolfram Research. Last Modified 2022. https://reference.wolfram.com/language/ref/NetInitialize.html.
APA
Wolfram Language. (2016). NetInitialize. Wolfram Language & System Documentation Center. Retrieved from https://reference.wolfram.com/language/ref/NetInitialize.html