CholeskyDecomposition
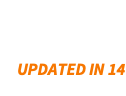
gives the Cholesky decomposition of a matrix m.
Details and Options

- The matrix m can be numerical or symbolic, but must be Hermitian and positive definite.
- CholeskyDecomposition[m] yields an upper‐triangular matrix u so that ConjugateTranspose[u].u==m.
- With the setting TargetStructure->"Structured", CholeskyDecomposition[m] returns u as an UpperTriangularMatrix.
Examples
open allclose allBasic Examples (2)
Scope (11)
Basic Uses (7)
Find the Cholesky decomposition of a machine-precision matrix:
Cholesky decomposition for a complex matrix:
CholeskyDecomposition will detect if the input fails to be Hermitian and positive definite:

The matrix m is positive definite but not Hermitian:
Use CholeskyDecomposition with an exact matrix:
Cholesky decomposition for an arbitrary-precision matrix:
Use CholeskyDecomposition with a symbolic matrix:
When verifying the result, conditions are needed to make sure the matrix is positive definite:
This can be understood as making sure all eigenvalues are positive:
The Cholesky decomposition for a large numerical matrix is computed efficiently:
Cholesky decomposition for a real symmetric positive definite CenteredInterval matrix:
Special Matrices (4)
Find the Cholesky decomposition for sparse matrices:
Cholesky decompositions of structured matrices:
Use with a QuantityArray structured matrix:
Cholesky decomposition of an identity matrix is an identity matrix:
Cholesky decomposition of HilbertMatrix:
Options (1)
TargetStructure (1)
With TargetStructure->"Dense", the result is returned as a dense matrix:
With TargetStructure->"Structured", the result is returned as an UpperTriangularMatrix:
Applications (2)
A triangular linear system is a system of linear equations in which the first equation has one variable and each subsequent equation introduces exactly one additional variable. Rewrite the following system in three variables as two triangular linear systems in six variables:
Rewrite the system in matrix form and compute the Cholesky decomposition of
:
The original system can be reordered into :
Introduce new variables and set
; the result is a triangular system in
:
Substituting into
gives
, a triangular system in
:
The six triangular equations together give the same result for as the original system of equations:
The Cholesky decomposition can be used to create random samples having a specified covariance from many independent random values, for example, in Monte Carlo simulation. Starting from the desired covariance matrix, compute the lower triangular matrix , where
is the Cholesky decomposition:
Generate a million independent samples and multiply each one by :
The covariance of the sample agrees with the desired covariance to roughly three digits:
Properties & Relations (6)
The Cholesky decomposition requires the input matrix to be Hermitian and positive definite:
Verify ConjugateTranspose[u].u == m:
CholeskyDecomposition[m] is upper triangular and positive definite:
The Cholesky decomposition is not the same thing as the matrix square root:
The Cholesky decomposition is upper triangular, whereas the square root is Hermitian:
However, both matrices are positive definite and have the same determinant:
For a real matrix , the Cholesky decomposition of
coincides with
's QR decomposition up to sign:
Find the Cholesky decomposition of Transpose[m].m:
Compute QRDecomposition[m]:
is the same as
except for the choice of sign for each row:
For any matrix , the Cholesky decomposition of
coincides with
's QR decomposition up to phase:
Find the Cholesky decomposition of ConjugateTranspose[m].m:
Compute QRDecomposition[m]:
is the same as
except for the choice of phase for each row:
CholeskyDecomposition is a kind of LU decomposition:
This is generally a different decomposition from the one given by LUDecomposition:
Possible Issues (2)
Matrices need to be positive definite enough to overcome numerical roundoff:

The smallest eigenvalue is effectively zero to machine precision:
The decomposition can be computed when the precision is high enough to resolve it:
s is a sparse tridiagonal matrix:
The Cholesky decomposition is computed as a dense matrix even if the result is sparse:
Using LinearSolve will give a LinearSolveFunction that has a sparse Cholesky factorization:
Text
Wolfram Research (2003), CholeskyDecomposition, Wolfram Language function, https://reference.wolfram.com/language/ref/CholeskyDecomposition.html (updated 2024).
CMS
Wolfram Language. 2003. "CholeskyDecomposition." Wolfram Language & System Documentation Center. Wolfram Research. Last Modified 2024. https://reference.wolfram.com/language/ref/CholeskyDecomposition.html.
APA
Wolfram Language. (2003). CholeskyDecomposition. Wolfram Language & System Documentation Center. Retrieved from https://reference.wolfram.com/language/ref/CholeskyDecomposition.html