PageRankCentrality
PageRankCentrality[g,α]
gives a list of page-rank centralities for the vertices in the graph g and weight α.
PageRankCentrality[g,α,β]
gives a list of page-rank centralities, using weight α and initial centralities β.
PageRankCentrality[{vw,…},…]
uses rules vw to specify the graph g.
Details and Options
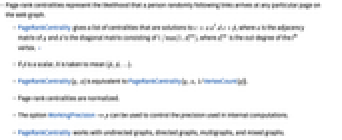
- Page-rank centralities represent the likelihood that a person randomly following links arrives at any particular page on the web graph.
- PageRankCentrality gives a list of centralities that are solutions to
, where
is the adjacency matrix of g and
is the diagonal matrix consisting of
, where
is the out-degree of the
vertex. »
- If β is a scalar, it is taken to mean {β,β,…}.
- PageRankCentrality[g,α] is equivalent to PageRankCentrality[g,α,1/VertexCount[g]].
- Page-rank centralities are normalized.
- The option WorkingPrecision->p can be used to control the precision used in internal computations.
- PageRankCentrality works with undirected graphs, directed graphs, multigraphs, and mixed graphs.
Examples
open allclose allBasic Examples (2)
Scope (7)
PageRankCentrality works with undirected graphs:
Use rules to specify the graph:
Nondefault initial centralities:
PageRankCentrality works with large graphs:
Options (3)
WorkingPrecision (3)
By default, PageRankCentrality finds centralities using machine-precision computations:
Specify a higher working precision:
Infinite working precision corresponds to exact computation:
Applications (6)
Rank websites based on the likelihood that a person randomly clicking on hyperlinks will reach a particular page:
Highlight the page-rank centrality for CycleGraph:
A corporate network of web pages linked via hyperlinks. Find the page that you are most likely to arrive at after a large number of clicks, with a damping factor of 0.85:
A road network where a node represents a road, and two roads are connected if they intersect. Predict the road that always has a traffic flow:
Find species whose extinctions would lead to ecosystem collapse in a food chain:
A metabolic cellular network for Neisseria gonorrhoeae. Find those proteins that play a marginal functional role in the system:
Properties & Relations (3)
The centrality vector is the normalized solution of the linear system :
Page-rank centralities are normalized:
Use VertexIndex to obtain the centrality of a specific vertex:
Text
Wolfram Research (2010), PageRankCentrality, Wolfram Language function, https://reference.wolfram.com/language/ref/PageRankCentrality.html (updated 2015).
CMS
Wolfram Language. 2010. "PageRankCentrality." Wolfram Language & System Documentation Center. Wolfram Research. Last Modified 2015. https://reference.wolfram.com/language/ref/PageRankCentrality.html.
APA
Wolfram Language. (2010). PageRankCentrality. Wolfram Language & System Documentation Center. Retrieved from https://reference.wolfram.com/language/ref/PageRankCentrality.html