AttentionLayer
represents a trainable net layer that learns to pay attention to certain portions of its input.
AttentionLayer[net]
specifies a particular net to give scores for portions of the input.
AttentionLayer[net,opts]
includes options for weight normalization, masking and other parameters.
Details and Options
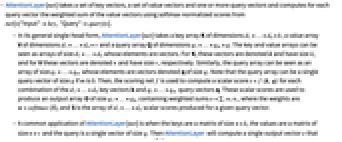
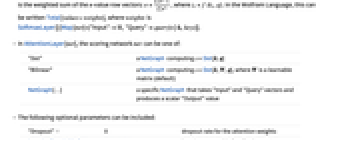
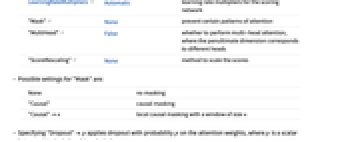
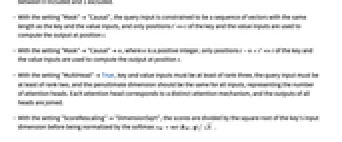
- AttentionLayer[net] takes a set of key vectors, a set of value vectors and one or more query vectors and computes for each query vector the weighted sum of the value vectors using softmax-normalized scores from net[<"Input"key,"Query"query >].
- In its general single-head form, AttentionLayer[net] takes a key array K of dimensions d1×…×dn×k, a value array V of dimensions d1×…×dn×v and a query array Q of dimensions q1×…×qm×q. The key and value arrays can be seen as arrays of size d1×…×dn whose elements are vectors. For K, these vectors are denoted k and have size k, and for V these vectors are denoted v and have size v, respectively. Similarly, the query array can be seen as an array of size q1×…×qm whose elements are vectors denoted q of size q. Note that the query array can be a single query vector of size q if m is 0. Then, the scoring net f is used to compute a scalar score s=f(k,q) for each combination of the d1×…×dn key vectors k and q1×…×qm query vectors q. These scalar scores are used to produce an output array O of size q1×…×qm containing weighted sums o=
wivi, where the weights are w=softmax(S), and S is the array of d1×…×dn scalar scores produced for a given query vector.
- A common application of AttentionLayer[net] is when the keys are a matrix of size n×k, the values are a matrix of size n×v and the query is a single vector of size q. Then AttentionLayer will compute a single output vector o that is the weighted sum of the n-value row vectors: o=
, where zi=f(ki,q). In the Wolfram Language, this can be written Total[values*weights], where weights is SoftmaxLayer[][Map[net[<"Input"#,"Query"query >]&,keys]].
- In AttentionLayer[net], the scoring network net can be one of:
-
"Dot" a NetGraph computing s=Dot[k,q] "Bilinear" a NetGraph computing s=Dot[k,W,q], where W is a learnable matrix (default) NetGraph[…] a specific NetGraph that takes "Input" and "Query" vectors and produces a scalar "Output" value - The following optional parameters can be included:
-
"Dropout" 0 dropout rate for the attention weights LearningRateMultipliers Automatic learning rate multipliers for the scoring network "Mask" None prevent certain patterns of attention "MultiHead" False whether to perform multi-head attention, where the penultimate dimension corresponds to different heads "ScoreRescaling" None method to scale the scores - Possible settings for "Mask" are:
-
None no masking "Causal" causal masking "Causal"n local causal masking with a window of size n - Specifying "Dropout"p applies dropout with probability p on the attention weights, where p is a scalar between 0 included and 1 excluded.
- With the setting "Mask""Causal", the query input is constrained to be a sequence of vectors with the same length as the key and the value inputs, and only positions t'<=t of the key and the value inputs are used to compute the output at position t.
- With the setting "Mask""Causal"n, where n is a positive integer, only positions t-n<t'<=t of the key and the value inputs are used to compute the output at position t.
- With the setting "MultiHead"True, key and value inputs must be at least of rank three, the query input must be at least of rank two, and the penultimate dimension should be the same for all inputs, representing the number of attention heads. Each attention head corresponds to a distinct attention mechanism, and the outputs of all heads are joined.
- With the setting "ScoreRescaling""DimensionSqrt", the scores are divided by the square root of the key's input dimension before being normalized by the softmax:
.
- AttentionLayer is typically used inside NetGraph.
- AttentionLayer exposes the following input ports for use in NetGraph etc.:
-
"Key" an array of size d1×…×dn×k (or d1×…×dn×h×k with multi-head attention) "Value" an array of size d1×…×dn×v (or d1×…×dn×h×k with multi-head attention) "Query" an array of size q1×…×qm×q (or q1×…×qm×h×k with multi-head attention) - AttentionLayer exposes an output port for use in NetGraph etc.:
-
"Output" an array of outputs with dimensions q1×…×qm×v (or q1×…×qm×h×v with multi-head attention) - AttentionLayer exposes an extra port to access internal attention weights:
-
"AttentionWeights" an array of weights with dimensions d1×…×dn×q1×…×qm (or d1×…×dn×h×q1×…×qm with multi-head attention) - AttentionLayer[…,"Key"shape1,"Value"shape2,"Query"shape3] allows the shapes of the inputs to be specified. Possible forms for shapei include:
-
NetEncoder[…] encoder producing a sequence of arrays {d1,d2,…} an array of dimensions d1×d2×… {"Varying",d1,d2,…} an array whose first dimension is variable and remaining dimensions are d2×d3×… {Automatic,…} an array whose dimensions are to be inferred {"Varying",Automatic,…} a varying number of arrays each of inferred size - The sizes of the key, value and query arrays are usually inferred automatically within a NetGraph.
- AttentionLayer[…][<"Key"key,"Value"value,"Query"query >] explicitly computes the output from applying the layer.
- AttentionLayer[…][<"Key"{key1,key2,…},"Value"{value1,value2,…},"Query"{query1,query2,…} >] explicitly computes outputs for each of the keyi, valuei and queryi in a batch of inputs.
- AttentionLayer[…][input,NetPort["AttentionWeights"]] can be used to access the softmax-normalized attention weights on some input.
- When given a NumericArray in the input, the output will be a NumericArray.
- NetExtract[…,"ScoringNet"] can be used to extract net from an AttentionLayer[net] object.
- Options[AttentionLayer] gives the list of default options to construct the layer. Options[AttentionLayer[…]] gives the list of default options to evaluate the layer on some data.
- Information[AttentionLayer[…]] gives a report about the layer.
- Information[AttentionLayer[…],prop] gives the value of the property prop of AttentionLayer[…]. Possible properties are the same as for NetGraph.
Examples
open allclose allBasic Examples (2)
Create an AttentionLayer:
Create a randomly initialized AttentionLayer that takes a sequence of two-dimensional keys, three-dimensional values and a sequence of one-dimensional queries:
The layer threads across a batch of sequences of different lengths:
Scope (4)
Scoring Net (2)
Create an AttentionLayer using a "Dot" scoring net:
Extract the "Dot" scoring net:
Create a new AttentionLayer explicitly specifying the scoring net as a NetGraph object:
Create a custom scoring net with trainable parameters:
Create and initialize an AttentionLayer that makes use of the custom scoring net:
Attention Weights (2)
Create an AttentionLayer:
Compute attention weights on a given input:
In this case, the weights correspond to:
Compute both attention weights and outputs of the layer:
Take a model based on AttentionLayer:
This net contains several multi-head self-attention layers with 12 heads, for instance:
Extract the attention weights of this layer for a given input to the net:
Represent the weights of the first attention head as connection strengths between input tokens:
Options (7)
"Dropout" (1)
Define an AttentionLayer with dropout on attention weights masking:
Without training-specific behavior, the layer returns the same result as without dropout:
With NetEvaluationMode"Train", the layer returns different results:
LearningRateMultipliers (1)
Make a scoring net with arbitrary weights:
Use this scoring net in AttentionLayer, freezing its weights with the option LearningRateMultipliers:
A zero learning rate multiplier will be used for the weights of the scoring net when training:
"Mask" (2)
Define an AttentionLayer with causal masking:
Apply the attention layer with one query vector and a sequence of length five:
The output at a given step depends only on the keys and the values up to this step. In particular, the first output vector is the first vector of values.
The attention weights form a lower-triangular matrix:
Define an AttentionLayer with local causal masking of window size 3:
Apply the attention layer with one query vector and a sequence of length five:
The output at a given step depends only on the keys and the values from the last three steps.
This can be seen in the matrix of attention weights that contains zeros:
"MultiHead" (2)
Define an AttentionLayer with two heads:
Apply multi-head attention on one query vector and a sequence of length three:
The result is the same as applying single-head attention separately on each head and joining the result:
Define a NetGraph to perform multi-head self-attention with six heads:
Apply to a NumericArray with a sequence of length three:
"ScoreRescaling" (1)
Create an AttentionLayer that rescales attention scores with respect to the input dimension:
Evaluate the layer on an input:
The output is less contrasted than without score rescaling:
The attention weights are also less contrasted, even if their ordering remains the same:
Applications (1)
To sort lists of numbers, generate a test and training set consisting of lists of integers between 1 and 6:
Display three random samples drawn from the training set:
Define a NetGraph with an AttentionLayer:
Properties & Relations (4)
If the query, key and value inputs are matrices, AttentionLayer[net] computes:
Define an AttentionLayer and extract the scoring subnet:
Evaluate AttentionLayer on some test data:
AttentionLayer[net,"ScoreRescaling""DimensionSqrt"] computes:
Define an AttentionLayer and extract the scoring subnet:
Evaluate AttentionLayer on some test data:
AttentionLayer[scorer,"Mask""Causal","ScoreRescaling""DimensionSqrt"] computes:
Define an AttentionLayer and extract the scoring subnet:
Evaluate AttentionLayer on some test data:
If "Key" and "Value" inputs are the same, AttentionLayer is equivalent to the deprecated SequenceAttentionLayer:

Possible Issues (1)
When using the setting "Dot" for the scoring net net in AttentionLayer[net], the input key and query vectors cannot be different sizes:

This restriction does not apply to using a "Bilinear" scoring net:
Text
Wolfram Research (2019), AttentionLayer, Wolfram Language function, https://reference.wolfram.com/language/ref/AttentionLayer.html (updated 2022).
CMS
Wolfram Language. 2019. "AttentionLayer." Wolfram Language & System Documentation Center. Wolfram Research. Last Modified 2022. https://reference.wolfram.com/language/ref/AttentionLayer.html.
APA
Wolfram Language. (2019). AttentionLayer. Wolfram Language & System Documentation Center. Retrieved from https://reference.wolfram.com/language/ref/AttentionLayer.html