LeastSquares

LeastSquares[m,b]
finds an x that solves the linear least-squares problem for the matrix equation m.x==b.
LeastSquares[a,b]
finds an x that solves the linear least-squares problem for the array equation a.x==b.
Details and Options
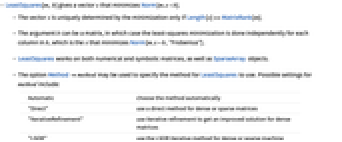
- LeastSquares[m,b] gives a vector x that minimizes Norm[m.x-b].
- The vector x is uniquely determined by the minimization only if Length[x]==MatrixRank[m].
- The argument b can be a matrix, in which case the least-squares minimization is done independently for each column in b, which is the x that minimizes Norm[m.x-b,"Frobenius"].
- LeastSquares works on both numerical and symbolic matrices, as well as SparseArray objects.
- For an n1×…×nk×m array a and an n1×…×nk×d1×…×dl array b, LeastSquares[a,b] gives an m×d1×…×dl array x, which minimizes Norm[Flatten[a.x-b]].
- The option Methodmethod may be used to specify the method for LeastSquares to use. Possible settings for method include:
-
Automatic choose the method automatically "Direct" use a direct method for dense or sparse matrices "IterativeRefinement" use iterative refinement to get an improved solution for dense matrices "LSQR" use the LSQR iterative method for dense or sparse machine number matrices "Krylov" use an iterative method for sparse machine number matrices
Examples
open allclose allBasic Examples (2)
Solve a simple least-squares problem:
This finds a tuple that minimizes
:
Use LeastSquares to minimize :
Compare to general minimization:
Note there is no solution to , so
may be regarded as the best approximate solution:

Scope (12)
Basic Uses (7)
Find the least squares for a machine-precision matrix:
Least squares for a complex matrix:
Use LeastSquares for an exact non-square matrix:
Least squares for an arbitrary-precision matrix:
Use LeastSquares with a symbolic matrix:
The least squares for a large numerical matrix is computed efficiently:
In LeastSquares[m,b], b can be a matrix:
Each column in the result equals the solution found by using the corresponding column in b as input:
Special Matrices (4)
Solve a least-squares problem for a sparse matrix:
Solve the least-squares problem with structured matrices:
Use a different type of matrix structure:
LeastSquares[IdentityMatrix[n],b] gives the vector :
Least squares of HilbertMatrix:
Options (1)
Tolerance (1)
m is a 20×20 Hilbert matrix, and b is a vector such that the solution of m.x==b is known:
With the default tolerance, numerical roundoff is limited, so errors are distributed:
With Tolerance->0, numerical roundoff can introduce excessive error:
Specifying a higher tolerance will limit roundoff errors at the expense of a larger residual:
Applications (9)
Geometry of Least Squares (4)
LeastSquares[m,b] can be understood as finding the solution to , where
is the orthogonal projection of
onto the column space of
. Consider the following
and
:
Find an orthonormal basis for the space spanned by the columns of :
Compute the orthogonal projection of
onto the spaced spanned by the
:
Visualize , its projections
onto the
, and
:
This is the same result as given by LeastSquares:
Compare and explain the answers returned by LeastSquares[m,b] and LinearSolve[m,b⟂] for the following and
:
Find an orthonormal basis for the space spanned by the columns of :
A zero vector is returned because the rank of the matrix is less than the number of columns:
Compute the orthogonal projection of
onto the spaced spanned by the
:
Find the solution returned by LeastSquares:
While x and xPerp are different, both solve the least-squares problem because m.x==m.xPerp:
The two solutions differ by an element of NullSpace[m]:
Use the matrix projection operators for a matrix with linearly independent columns to find LeastSquares[m,b] for the following and
:
The projection operator on the column space of is
:
The solution to the least-squares problem is then the unique solution to :
Confirm using LeastSquares:
Compare the solutions found using LeastSquares[m,b] and LinearSolve together with the normal equations of and for the following
and
:
Solve using LeastSquares:
Solve using LinearSolve and the normal equations :
While x and xNormal are different, both solve the least-squares problem because m.x==m.xNormal:
The two solutions differ by an element of NullSpace[m]:
Curve and Parameter Fitting (5)
LeastSquares can be used to find a best-fit curve to data. Consider the following data:
Extract the and
coordinates from the data:
Let have the columns
and
, so that minimizing
will be fitting to a line
:
Get the coefficients and
for a linear least‐squares fit:
Verify the coefficients using Fit:
Plot the best-fit curve along with the data:
Find the best-fit parabola to the following data:
Extract the and
coordinates from the data:
Let have the columns
,
and
, so that minimizing
will be fitting to
:
Get the coefficients ,
and
for a least‐squares fit:
Verify the coefficients using Fit:
Plot the best-fit curve along with the data:
A healthy child’s systolic blood pressure (in millimeters of mercury) and weight
(in pounds) are approximately related by the equation
. Use the following experimental data points
to estimate the systolic blood pressure of a healthy child weighing 100 pounds:
Use DesignMatrix to construct the matrix with columns and
:
Extract the values from the data:
The least-squares solution of :
Substitute the parameters into the model:
Then the expected blood pressure of a child weighing 100 pounds is roughly :
Visualize the best-fit curve and the data:
According to Kepler’s first law, a comet's orbit satisfies , where
is a constant and
is the eccentricity. The eccentricity determines the type of orbit, with
for an ellipse,
for a parabola, and
for a hyperbola. Use the following observational data to determine the type of orbit of the comet and predict its distance from the Sun at
:
To find and
, first use DesignMatrix to create the matrix whose columns are
and
:
Use LeastSquares to find the and
that minimize the error in
from the design matrix:
Since , the orbit is elliptical and there is a unique value of
for each value of
:
Evaluating the function at gives an expected distance of roughly
:
Extract the and
coordinates from the data:
Define cubic basis functions centered at t with support on the interval [t-2,t+2]:
Set up a sparse design matrix for basis functions centered at 0, 1, ..., 10:
Solve the least-squares problem:
Visualize the data with the best-fit piecewise cubic, which is :
Properties & Relations (12)
If m.x==b can be solved, LeastSquares is equivalent to LinearSolve:
If x=LeastSquares[m,b] and n lies in NullSpace[m], x+n is also a least-squares solution:
LeastSquares[m,b] solves , with
the orthogonal projection onto the columns of
:
Equality was guaranteed because this particular matrix has a trivial null space:
If is real valued, x=LeastSquares[m,b] obeys the normal equations
:
For a complex-valued matrix, the equations are :
Given x==LeastSquares[m,b], m.x-b lies in NullSpace[ConjugateTranspose[m]]:
The null space is two dimensional:
m.ls-b lies in the span for the two vectors, as expected:
LeastSquares and PseudoInverse can both be used to solve the least-squares problem:
LeastSquares and QRDecomposition can both be used to solve the least-squares problem:
Let m be a matrix with an empty nullspace:
For a vector b, LeastSquares[m,b] is equivalent to ArgMin[Norm[m.x-b],x]:
It is also equivalent to ArgMin[Norm[m.x-b,"Frobenius"],x]:
Let m be a matrix with an empty nullspace:
For a matrix b, LeastSquares is equivalent to ArgMin[Norm[m.x-b,"Frobenius"],x]:
If b is a matrix, each column in LeastSquares[m,b] is the result for the corresponding column in b:
m is a 5×2 matrix, and b is a length-5 vector:
Solve the least-squares problem:
It is also gives the coefficients for the line with least-squares distance to the points:
LeastSquares gives the parameter estimates for a linear model with normal errors:
LinearModelFit fits the model and gives additional information about the fitting:
Text
Wolfram Research (2007), LeastSquares, Wolfram Language function, https://reference.wolfram.com/language/ref/LeastSquares.html (updated 2024).
CMS
Wolfram Language. 2007. "LeastSquares." Wolfram Language & System Documentation Center. Wolfram Research. Last Modified 2024. https://reference.wolfram.com/language/ref/LeastSquares.html.
APA
Wolfram Language. (2007). LeastSquares. Wolfram Language & System Documentation Center. Retrieved from https://reference.wolfram.com/language/ref/LeastSquares.html