DimensionReduction
DimensionReduction[{example1,example2,…}]
生成从 examplei 定义的空间投影到低维近似流形的 DimensionReducerFunction[…].
DimensionReduction[examples,n]
对 n 维近似流形生成 DimensionReducerFunction[…].
DimensionReduction[examples,n,props]
生成维数降低的指定属性.
更多信息和选项
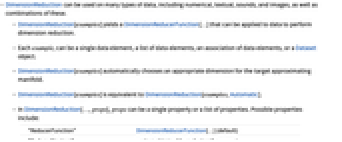
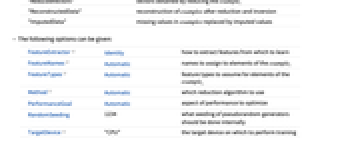
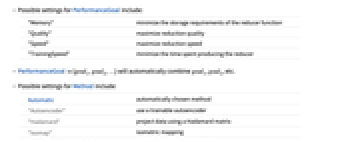
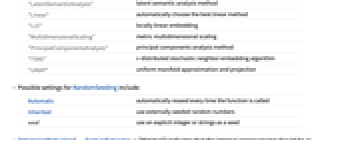
- DimensionReduction 可用于许多数据类型,包括数值、文本、声音和图像以及它们的组合.
- DimensionReduction[examples] 生成 DimensionReducerFunction[…] ,可应用于数据以执行降维.
- 每个 examplei 可以是单个数据元素、数据元素列表、数据元素关联或 Dataset 对象.
- DimensionReduction[examples] 自动选择与目标近似流形相应的维度.
- DimensionReduction[examples] 等价于 DimensionReduction[examples,Automatic].
- 在 DimensionReduction[…,props] 中,props 可以是单一属性或者是属性列表. 可能的属性包括:
-
"ReducerFunction" DimensionReducerFunction[…] (默认) "ReducedVectors" 通过把 examplei 降维获得的向量 "ReconstructedData" 降维和反演后重建 examples "ImputedData" 用插补值替换 examples 中的缺失值 - 可以给出以下选项:
-
FeatureExtractor Identity 怎样提取要学习的特征 FeatureNames Automatic 分配给 examplei 的元素的名称 FeatureTypes Automatic 假设 examplei 的元素的特征类型 Method Automatic 要使用哪种降维算法 PerformanceGoal Automatic 优化的性能方面 RandomSeeding 1234 内部应怎样对伪随机数字生成器进行播种 TargetDevice "CPU" 执行培训的目标设备 - PerformanceGoal 的可能设置包括:
-
"Memory" 降维函数的存储要求最小化 "Quality" 降维质量最大化 "Speed" 降维速度最大化 "TrainingSpeed" 生成降维器所用时间最小化 - PerformanceGoal{goal1,goal2,…} 将自动合并 goal1、goal2 等.
- Method 的可能设置包括:
-
Automatic 自动选择方法 "Autoencoder" 使用可训练的自动编码器 "Hadamard" 用 Hadamard 矩阵对数据进行投影 "Isomap" 等距映射 "LatentSemanticAnalysis" 潜在语义分析方法 "Linear" 自动选择最佳的线性方法 "LLE" 局部线性嵌入 "MultidimensionalScaling" 度量多维尺度分析 (metric multidimensional scaling) "PrincipalComponentsAnalysis" 主成分分析方法 "TSNE" -分布随机领域嵌入算法
"UMAP" 统一流形逼近与投影 (uniform manifold approximation and projection) - RandomSeeding 的可能设置包括:
-
Automatic 每次调用函数时都自动重新播种 Inherited 使用外部播种的随机数字 seed 明确指定整数或字符串作为种子 - DimensionReduction[…,FeatureExtractor"Minimal"] 表明内部预处理应尽量简单.
- DimensionReduction[DimensionReducerFunction[…],FeatureExtractorfe] 可用于预置 FeatureExtractorFunction[…] fe 于已存在的特征提取器.
范例
打开所有单元关闭所有单元基本范例 (3)
范围 (7)
对 DateObject 列表培训降维器:
减少新的 DateObject 的维数:
选项 (7)
FeatureTypes (1)
第一个特征被解释为数值. 使用 FeatureTypes 加强第一个特征解释为标称值:
Method (3)
TargetDevice (1)
使用在系统默认 GPU 上的完全连接的 "AutoEncoder" 培训约简函数并查看 AbsoluteTiming:
应用 (5)
数据集可视化 (1)
头部姿势估计 (1)
图像插补 (1)
从 ExampleData 加载 MNIST 数据集,并保留图像:
用 Missing[] 替换向量的某些值,并可视化:
推荐系统 (1)
得到 SparseArray 形式的用户影评:
数据集由 100 个用户和 10 部影片组成. 评分范围从 1 到 5,并用 Missing[] 表示未知评分:
图像搜索 (1)
在降维空间产生一个 NearestFunction:
使用 NearestFunction,构建显示数据集最近图像的函数:
文本
Wolfram Research (2015),DimensionReduction,Wolfram 语言函数,https://reference.wolfram.com/language/ref/DimensionReduction.html (更新于 2020 年).
CMS
Wolfram 语言. 2015. "DimensionReduction." Wolfram 语言与系统参考资料中心. Wolfram Research. 最新版本 2020. https://reference.wolfram.com/language/ref/DimensionReduction.html.
APA
Wolfram 语言. (2015). DimensionReduction. Wolfram 语言与系统参考资料中心. 追溯自 https://reference.wolfram.com/language/ref/DimensionReduction.html 年