ParameterMixtureDistribution
ParameterMixtureDistribution[dist[θ],θwdist]
represents a parameter mixture distribution where the parameter θ is distributed according to the weight distribution wdist.
ParameterMixtureDistribution[dist[θ1,θ2,…],{θ1wdist1,θ2wdist2,…}]
represents a parameter mixture distribution where the parameter θ1 has weight distribution wdist1, θ2 has weight distribution wdist2, etc.
Details and Options
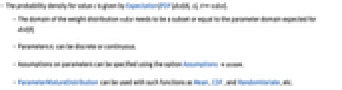
- The probability density for value
is given by Expectation[PDF[dist[θ],x],θwdist].
- The domain of the weight distribution wdist needs to be a subset or equal to the parameter domain expected for dist[θ].
- Parameters θi can be discrete or continuous.
- Assumptions on parameters can be specified using the option Assumptionsassum.
- ParameterMixtureDistribution can be used with such functions as Mean, CDF, and RandomVariate, etc.
Examples
open allclose allBasic Examples (3)
Scope (36)
Basic Uses (7)
Find the parameter mixture of ExponentialDistribution with uniformly distributed weight:
The weight domain must satisfy the parameter assumptions of the main distribution:
Pick a weight distribution supported on the positive reals:
The resulting parameter mixture CDF:
Use a discrete weight for a discrete parameter:
Compare the probability density functions:
Compare probabilities of obtaining a value less than 4 from each distribution:
Cumulative distribution function:
Use a multivariate distribution as a weight to vary more than one parameter:
Use more than one weight distribution to vary multiple parameters:
Visualize the probability density function:
Estimate parameters in a parameter mixture distribution:
Create a random sample for a choice of weight distribution parameters:
Find the distribution parameters:
Compare the histogram of the sample with the PDF of the estimated distribution:
Weight Distributions (5)
Define a parameter mixture with a continuous univariate weight distribution:
Define a parameter mixture with a discrete univariate weight distribution:
Find the probability density function:
Moment has closed form for a symbolic order:
Define a parameter mixture with a discrete univariate weight distribution:
Define a parameter mixture with a multivariate weight distribution:
Verify that the integral of the PDF is 1:
Parametric Distributions (8)
Define a parameter mixture of a continuous univariate distribution:
Cumulative distribution function:
Find a mixture of a continuous univariate distribution:
Define a parameter mixture of a discrete univariate distribution:
Find a parameter mixture of a discrete univariate distribution:
For a fixed value of , Moment has closed form for symbolic order:
Define a parameter mixture of a bivariate continuous distribution:
Use a random sample and a smooth histogram to visualize the density function:
Define a parameter mixture of a bivariate distribution:
Find a parameter mixture distribution of a multivariate discrete distribution:
Histograms for each component:
LaplaceDistribution can be represented as a parametric mixture:
Nonparametric Distributions (3)
Use an EmpiricalDistribution as a weight:
Use a HistogramDistribution as a weight:
Define a parameter mixture with a SmoothKernelDistribution as a weight:
Derived Distributions (11)
Use a ProductDistribution as a weight distribution in a parameter mixture:
Use the histogram of a random sample from to visualize the PDF:
Find a parameter mixture distribution of a TransformedDistribution:
Visualize the density function using a random sample:
Find a parameter mixture using a TransformedDistribution as a weight distribution:
Use a MixtureDistribution as a weight distribution in a parameter mixture:
Vary weights in a MixtureDistribution according to a probability distribution:
Compare to the MixtureDistribution with fixed weights at the average values:
Both density functions are equal:
Define a parameter mixture of a TruncatedDistribution:
Define a parameter distribution of a TruncatedDistribution:
Visualize the probability density function using random sample:
Use a TruncatedDistribution as a weight distribution in a parameter mixture:
Find a parameter mixture distribution of an OrderDistribution:
Use an OrderDistribution as a weight distribution:
Parameter mixture of QuantityDistribution evaluates to QuantityDistribution:
Automatic Simplifications (2)
Discrete Distributions (1)
Parameter mixtures with BetaDistribution as a weight:
Parameter mixtures involving PoissonDistribution:
Continuous Distributions (1)
Options (1)
Assumptions (1)
WaringYuleDistribution is a parameter mixture of geometric distribution and UniformDistribution:
Applications (7)
SuzukiDistribution is defined as a parameter mixture of RayleighDistribution and LogNormalDistribution:
KDistribution can be represented as a parameter mixture of RayleighDistribution and GammaDistribution:
The time in minutes it takes a bank teller to serve a customer follows ExponentialDistribution, with average time having LindleyDistribution with mean 3. Find the service time distribution:
Simulate the service time for the next 30 customers:
In an optical communication system, transmitted light generates current at the receiver. The number of electrons follows the parametric mixture of a Poisson distribution and another distribution, depending on the type of light. If the source uses coherent laser light of intensity , then the electron-count distribution is Poisson:
Which is PoissonDistribution:
If the source uses thermal illumination, then the Poisson parameter follows ExponentialDistribution with parameter , and the electron count distribution can be determined:
These two distributions are distinguishable and allow you to determine the type of source:
The Voigt spectral line profile results from combining a Doppler (Cauchy) profile and a Lorentzian (normal) profile:
Compute the profile half-width:
Define modified normal distribution:
Plot modified normal distribution densities for several values of and compare them with standard normal density:
Properties & Relations (4)
The PDF of a parameter mixture can be computed using Expectation:
Parameter mixture with a discrete weight, assuming a finite number of values can be represented as a MixtureDistribution:
Parameter mixture with a discrete weight, assuming a countable number of values can be approximated by a MixtureDistribution:
Compare approximations for different quantiles as cut-offs:
Approximating a parameter mixture with a continuous weight by a MixtureDistribution:
Text
Wolfram Research (2010), ParameterMixtureDistribution, Wolfram Language function, https://reference.wolfram.com/language/ref/ParameterMixtureDistribution.html (updated 2016).
CMS
Wolfram Language. 2010. "ParameterMixtureDistribution." Wolfram Language & System Documentation Center. Wolfram Research. Last Modified 2016. https://reference.wolfram.com/language/ref/ParameterMixtureDistribution.html.
APA
Wolfram Language. (2010). ParameterMixtureDistribution. Wolfram Language & System Documentation Center. Retrieved from https://reference.wolfram.com/language/ref/ParameterMixtureDistribution.html