Classify
Listing of Built-in Classifiers »Classify[{in1class1,in2class2,…}]
generates a ClassifierFunction that attempts to predict classi from the example ini.
Classify[data,input]
attempts to predict the output associated with input from the training examples given.
Classify[data,input,prop]
computes the specified property prop relative to the prediction.
Details and Options
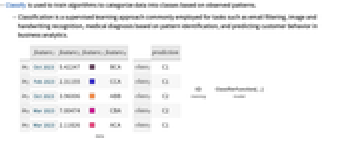
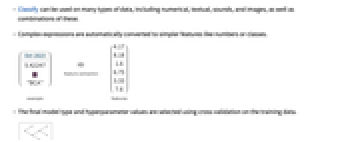
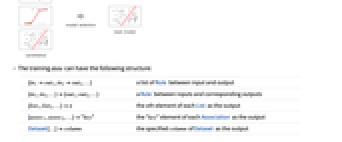
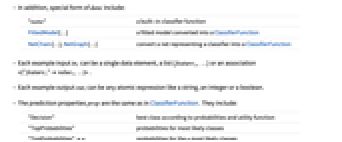
- Classify is used to train algorithms to categorize data into classes based on observed patterns.
- Classification is a supervised learning approach commonly employed for tasks such as email filtering, image and handwriting recognition, medical diagnosis based on pattern identification, and predicting customer behavior in business analytics.
- Classify can be used on many types of data, including numerical, textual, sounds, and images, as well as combinations of these.
- Complex expressions are automatically converted to simpler features like numbers or classes.
- The final model type and hyperparameter values are selected using cross-validation on the training data.
- The training data can have the following structure:
-
{in1out1,in2out2,…} a list of Rule between input and output {in1,in2,…}{out1,out2,…} a Rule between inputs and corresponding outputs {list1,list2,…}n the nth element of each List as the output {assoc1,assoc2,…}"key" the "key" element of each Association as the output Dataset[…]column the specified column of Dataset as the output - In addition, special form of data include:
-
"name" a built-in classifier function FittedModel[…] a fitted model converted into a ClassifierFunction NetChain[…],NetGraph[…] convert a net representing a classifier into a ClassifierFunction - Each example input ini can be a single data element, a list {feature1, …} or an association <"feature1"value1,… > .
- Each example output outi can be any atomic expression like a string, an integer or a boolean.
- The prediction properties prop are the same as in ClassifierFunction. They include:
-
"Decision" best class according to probabilities and utility function "TopProbabilities" probabilities for most likely classes "TopProbabilities"n probabilities for the n most likely classes "Probability"class probability for a specific class "Probabilities" association of probabilities for all possible classes "SHAPValues" Shapley additive feature explanations for each example "Properties" list of all properties available - "SHAPValues" assesses the contribution of features by comparing predictions with different sets of features removed and then synthesized. The option MissingValueSynthesis can be used to specify how the missing features are synthesized. SHAP explanations are given as odds ratio multipliers with respect to the class training prior. "SHAPValues"n can be used to control the number of samples used for the numeric estimations of SHAP explanations.
- Examples of built-in classifier functions include:
-
"CountryFlag" which country a flag image is for "FacebookTopic" which topic a Facebook post is about "FacialAge" estimated age from a face "FacialExpression" what type of expression a face displays "FacialGender" what gender a face appears to be "Language" which natural language text is in "LanguageExtended" language of a text, including rare languages "NameGender" which gender a first name is "NotablePerson" what notable person an image is of "NSFWImage" whether an image is considered "not safe for work" "Profanity" whether text contains profanity "ProgrammingLanguage" which programming language text is in "Sentiment" sentiment of a social media post "Spam" whether email is spam - The following options can be given:
-
AnomalyDetector None anomaly detector used by the classifier AcceptanceThreshold Automatic rarer-probability threshold for anomaly detector ClassPriors Automatic explicit prior probabilities for classes FeatureExtractor Identity how to extract features from which to learn FeatureNames Automatic feature names to assign for input data FeatureTypes Automatic feature types to assume for input data IndeterminateThreshold 0 below what probability to return Indeterminate Method Automatic which classification algorithm to use MissingValueSynthesis Automatic how to synthesize missing values PerformanceGoal Automatic aspects of performance to try to optimize RandomSeeding 1234 what seeding of pseudorandom generators should be done internally RecalibrationFunction Automatic how to post-process class probabilities TargetDevice "CPU" the target device on which to perform training TimeGoal Automatic how long to spend training the classifier TrainingProgressReporting Automatic how to report progress during training UtilityFunction Automatic utility as function of actual and predicted class ValidationSet Automatic the set of data on which to evaluate the model during training - Possible settings for Method include:
-
"ClassDistributions" classify using learned distributions "DecisionTree" classify using a decision tree "GradientBoostedTrees" classify using an ensemble of trees trained with gradient boosting "LogisticRegression" classify using probabilities from linear combinations of features "Markov" classify using a Markov model on the sequence of features (only for text, bag of token, etc.) "NaiveBayes" classify by assuming probabilistic independence of features "NearestNeighbors" classify from nearest neighbor examples "NeuralNetwork" classify using an artificial neural network "RandomForest" classify using Breiman–Cutler ensembles of decision trees "SupportVectorMachine" classify using a support vector machine - Using FeatureExtractor"Minimal" indicates that the internal preprocessing should be as simple as possible.
- Possible settings for PerformanceGoal include:
-
"DirectTraining" train directly on the full dataset, without model searching "Memory" minimize storage requirements of the classifier "Quality" maximize accuracy of the classifier "Speed" maximize speed of the classifier "TrainingSpeed" minimize time spent producing the classifier Automatic automatic tradeoff among speed, accuracy, and memory {goal1,goal2,…} automatically combine goal1, goal2, etc. - The following settings for TrainingProgressReporting can be used:
-
"Panel" show a dynamically updating graphical panel "Print" periodically report information using Print "ProgressIndicator" show a simple ProgressIndicator "SimplePanel" dynamically updating panel without learning curves None do not report any information - Possible settings for RandomSeeding include:
-
Automatic automatically reseed every time the function is called Inherited use externally seeded random numbers seed use an explicit integer or strings as a seed - In Classify[ClassifierFunction[…],FeatureExtractorfe], the FeatureExtractorFunction[…] fe will be prepended to the existing feature extractor.
- Information can be used on the ClassifierFunction[…] obtained.
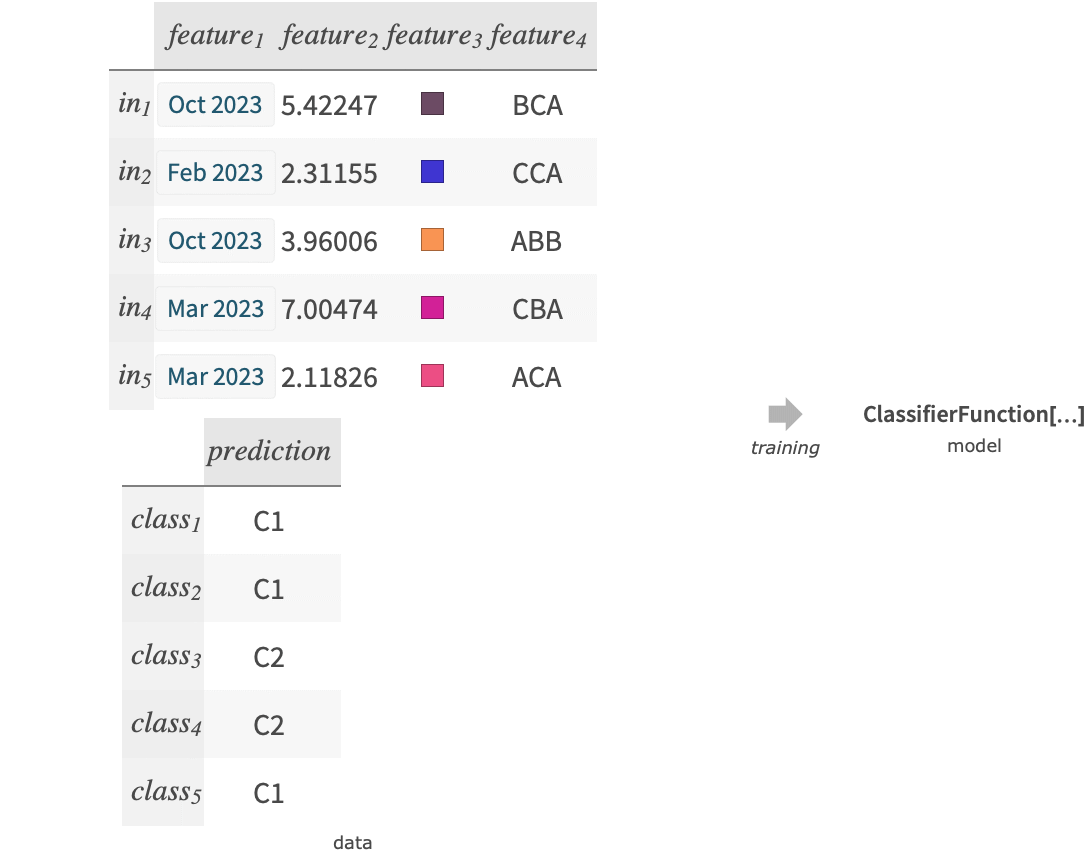
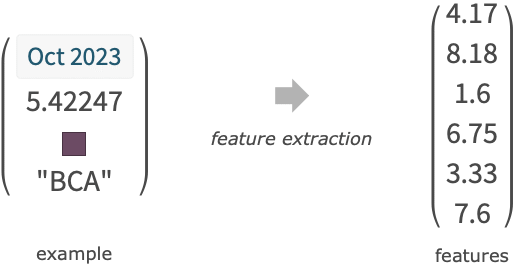
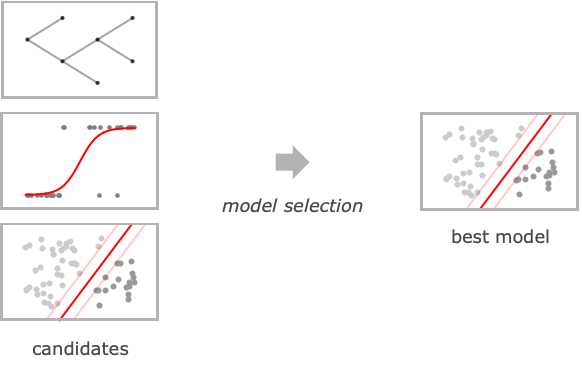
Examples
open allclose allBasic Examples (2)
Scope (32)
Data Format (7)
Specify the training set as a list of rules between an input example and the output value:
Each example can contain a list of features:
Each example can contain an association of features:
Specify the training set as a list of rules between a list of inputs and a list of outputs:
Specify all the data in a matrix and mark the output column:
Specify all the data in a list of associations and mark the output key:
Specify all the data in a dataset and mark the output column:
Data Types (13)
Numerical (3)
Nominal (3)
Quantities (1)
Train a classifier on data including Quantity objects:
Use the classifier on a new example:
Predict the most likely price when only the "Price" is known:
Information (4)
Built-in Classifiers (8)
Use the "Language" built-in classifier to detect the language in which a text is written:
Use it to detect the language of examples:
Obtain the probabilities for the most likely languages:
Restrict the classifier to some languages with the option ClassPriors:
Use the "FacebookTopic" built-in classifier to detect the topic of a Facebook post:
Unrecognized topics or languages will return Indeterminate:
Use the "CountryFlag" built-in classifier to recognize a country from its flag:
Use the "NameGender" built-in classifier to get the probable sex of a person from their first name:
Use the "NotablePerson" built-in classifier to determine what notable person is depicted in the given image:
Use the "Sentiment" built-in classifier to infer the sentiment of a social media message:
Use the "Profanity" built-in classifier to return True if a text contains strong language:
Use the "Spam" built-in classifier to detect if an email is spam from its content:
Options (23)
AcceptanceThreshold (1)
AnomalyDetector (1)
Create a classifier and specify that an anomaly detector should be included:
Evaluate the classifier on an non-anomalous input:
Evaluate the classifier on an anomalous input:
The "Probabilities" property is not affected by the anomaly detector:
Temporarily remove the anomaly detector from the classifier:
Permanently remove the anomaly detector from the classifier:
ClassPriors (1)
Train a classifier on an imbalanced dataset:
The training example 5False is classified as True:
Classify this example with a uniform prior over classes instead of the imbalanced training prior:
The class priors can be specified during the training:
The class priors of a classifier can also be changed after training:
FeatureExtractor (3)
Train a FeatureExtractorFunction on a simple dataset:
Use the feature extractor function as a preprocessing step in Classify:
Train a classifier on texts preprocessed by custom functions and an extractor method:
Create a feature extractor and extract features from a dataset of texts:
Train a classifier on the extracted features:
FeatureNames (2)
Train a classifier and give a name to each feature:
Use the association format to predict a new example:
The list format can still be used:
Train a classifier on a training set with named features and use FeatureNames to set their order:
FeatureTypes (2)
Train a classifier on data where the feature is intended to be a sequence of tokens:
Classify wrongly assumed that examples contained two different text features:
The following classification will output an error message:

Force Classify to interpret the feature as a "NominalSequence":
Train a classifier with named features:
Both features have been considered numerical:
Specify that the feature "gender" should be considered nominal:
IndeterminateThreshold (1)
Method (3)
Train a random forest classifier:
Plot the probability of class "a" given the feature for both classifiers:
Train a nearest neighbors classifier:
Find the classification accuracy on a test set:
In this example, using a naive Bayes classifier reduces the classification accuracy:
However, using a naive Bayes classifier reduces the classification time:
MONK's problems consist of synthetic binary classification datasets used for comparing the performance of different classifiers. Generate the dataset for the second MONK problem:
Test the accuracy of each available classifier by training on 169 examples and testing on the entire dataset:
MissingValueSynthesis (1)
Train a classifier with two input features:
Get class probabilities for an example that has a missing value:
Set the missing value synthesis to replace each missing variable with its estimated most likely value given known values (which is the default behavior):
Replace missing variables with random samples conditioned on known values:
Averaging over many random imputations is usually the best strategy and allows obtaining the uncertainty caused by the imputation:
Specify a learning method during training to control how the distribution of data is learned:
Classify an example with missing values using the "KernelDensityEstimation" distribution to condition values:
Provide an existing LearnedDistribution at training to use it when imputing missing values during training and later evaluations:
Specify an existing LearnedDistribution to synthesize missing values for an individual evaluation:
Control both the learning method and the evaluation strategy by passing an association at training:
RecalibrationFunction (1)
PerformanceGoal (1)
TargetDevice (1)
Train a classifier on the system's default GPU using a neural network and look at the AbsoluteTiming:
Compare the previous result with the one achieved by using the default CPU computation:
TimeGoal (2)
TrainingProgressReporting (1)
UtilityFunction (1)
By default, the most probable class is predicted:
This corresponds to the following utility specification:
Train a classifier that penalizes examples of class "yes" being misclassified as "no":
The classifier decision is different despite the probabilities being unchanged:
Specifying a utility function when classifying supersedes the utility function specified at training:
Applications (10)
Titanic Survival (2)
Load the "Titanic" dataset, which contains a list of Titanic passengers with their age, sex, ticket class, and survival:
Visualize a sample of the dataset:
Train a logistic classifier on this dataset:
Calculate the survival probability of a 10-year-old girl traveling in third class:
Plot the survival probability as a function of age for some combinations of "class" and "sex":
Train a classifier to predict a person's odds of surviving or dying in the Titanic crash:
Calculate the prior odds of a passenger dying:
Use the classifier to predict the odds of a person dying:
Get an explanation of how each feature multiplied the model's predicted odds of a class:
Compare the model's explanation of feature impact to the base rate odds:
Fisher's Iris (3)
Train a classifier on the Fisher iris dataset to predict the species of Iris:
Predict the species of Iris from a list of features:
Test the accuracy of the classifier on a test set:
Generate a confusion matrix of the classifier on this test set:
Train a classifier that classifies movie review snippets as "positive" or "negative":
Classify an unseen movie review snippet:
Test the accuracy of the classifier on a test set:
Import examples of the writings of Shakespeare, Oscar Wilde, and Victor Hugo to train a classifier:
Image Recognition (3)
Train a digit recognizer on 100 examples from the MNIST database of handwritten digits:
Use the classifier to recognize unseen digits:
Analyze probabilities of a misclassified example:
Train a classifier on 32 images of legendary creatures:
Use the classifier to recognize unseen creatures:
Feature Explanation (1)
Import images of handwritten digits and select the 3s, 5s, and 8s:
Visualize a few of the images:
Convert the images into their pixel values and separate their class:
Train a classifier to identify the digit by its individual pixel values:
Learn a simple distribution of the data that treats each pixel as independent (for speed purposes):
Use the "SHAPValues" property to estimate how each pixel in an example impacted the predicted class:
Take the Log to convert the "odds multiplier" SHAP values onto a scale centered at 0:
Look at the impact of each pixel weighted by its darkness by multiplying by the pixel values:
Visualize how the pixels increased (red) or decreased (blue) the model's confidence the digit was a 0 or 6:
Possible Issues (1)
The RandomSeeding option does not always guarantee reproducibility of the result:
Neat Examples (2)
Define and plot clusters sampled from normal distributions:
Blend colors to reflect the probability density of the different classes for each method:
Draw in the box to test a logistic classifier trained on the dataset ExampleData[{"MachineLearning","MNIST"}]:
Text
Wolfram Research (2014), Classify, Wolfram Language function, https://reference.wolfram.com/language/ref/Classify.html (updated 2021).
CMS
Wolfram Language. 2014. "Classify." Wolfram Language & System Documentation Center. Wolfram Research. Last Modified 2021. https://reference.wolfram.com/language/ref/Classify.html.
APA
Wolfram Language. (2014). Classify. Wolfram Language & System Documentation Center. Retrieved from https://reference.wolfram.com/language/ref/Classify.html